PrintSyst Automates AM Decision Making with AI (Includes Video)
The startup’s cloud-based artificial intelligence tool is designed to help companies adopting additive manufacturing make fast, easy decisions about what and how to 3D print.
“So much of 3D printing is still manual. Even a skilled engineer is going to spend at least 30 minutes on a new part understanding the best orientation, technology and material,” says Eitan Yona. For an industrial manufacturing company looking to adopt additive manufacturing (AM) for hundreds of parts, it could cost tens of thousands of dollars in engineering time over a year just to determine whether and how to 3D print each one.
PrintSyst, the company that Yona cofounded in 2018 with his brother, Itamar Yona, offers a possible solution for much faster decision-making. Its cloud-based PrintSyst Perfecter tool based on artificial intelligence (AI) allows engineers to quickly assess the viability of their 3D designs and get insight into suitable processes, appropriate materials and anticipated cost. The user can upload an STL file and within a matter of minutes receive a verdict of approve, decline or warning based on its printability.
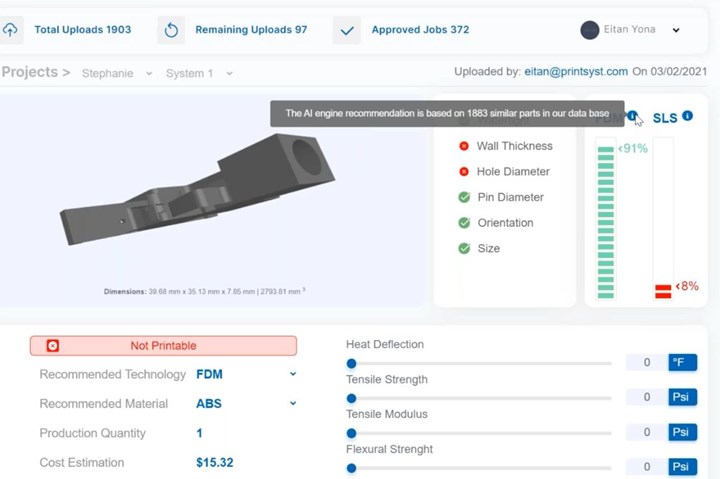
The PrintSyst engine has determined that this bracket is not printable in its current design, by comparing it to 1,883 similar parts in the AI’s database.
The Perfecter is able to issue this verdict by comparing each new uploaded part to other parts in its existing database. The more parts that are uploaded, the smarter the software becomes. (Defense and other sensitive applications can be kept separate from the main geometry database, however, in a private cloud or on-premise for security purposes.)
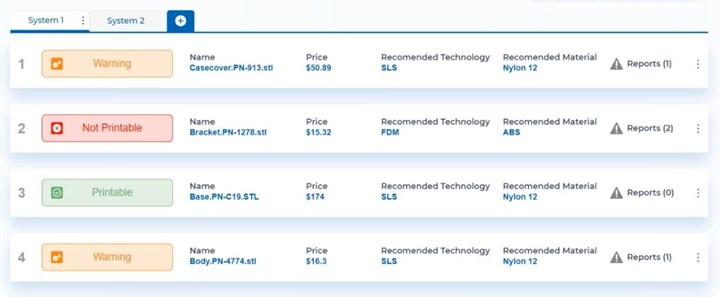
The AI is able to quickly analyze each part file to generate a verdict of Printable, Warning or Not Printable, allowing for faster decision making.
Whether a part is instantly approved or not, the user is presented with additional information and recommendations for the design. Any 3D printing process enabled in the user’s settings will be considered, and PrintSyst will make a suggestion based on the probability of success. A particular bracket, for instance, might have a 90% probability of success with fused filament fabrication (FFF), but only a 27% chance with selective laser sintering (SLS).
If problematic features such as thin walls are detected, the user will be prompted to adjust them with sliders to arrive at a more 3D printable design. Material recommendations are offered as well depending on the process, part requirements and user’s materials library, and a quote can be generated immediately based on the customizable rate settings. Within just a few minutes a PrintSyst user can go from STL to a 3D printable file with a price and probability of success.
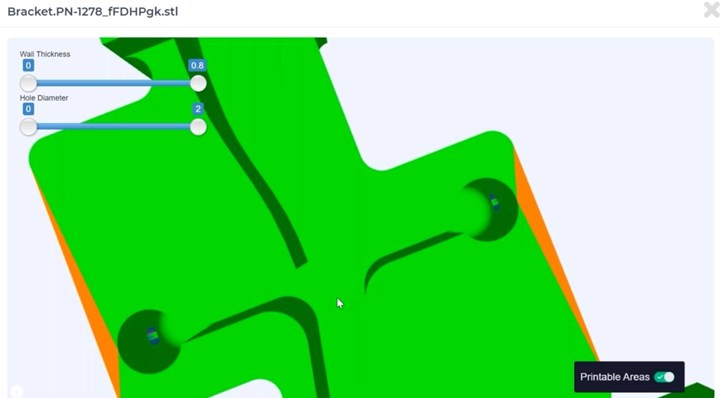
The software detects unprintable areas and gives the user guidance to adjust the geometry, reducing the time between design iterations.
That probability is growing more and more accurate in part because PrintSyst currently has knowledgeable AM users applying the software — humans with the ability to override its choices. Each time a user modifies or rejects the software’s suggestions, the AI learns. The same is true when users provide feedback on their success or failure with the build recommendations provided. The software becomes more reliable, “smarter,” over time as it encounters more parts and more users.
The result is a tool that allows manufacturers to make quick, easy and accurate decisions about 3D printing, Yona says. The process of adopting and implementing additive manufacturing properly can take months or years, and an AI-based engine like PrintSyst can reduce the startup time dramatically. Yet it’s not just about simplifying additive manufacturing in the present.
“We are working with AM experts to start,” Yona says, “but imagine that new employees come in and the existing ones retire. The knowledge management is still part of the engine. This software can make new employees experts immediately in terms of understanding materials, technology, parameters and orientation.”
The company’s current focus is working with industry leaders in aerospace, automotive and defense, but the PrintSyst Perfecter could be applied to any industrial manufacturing environment. The software will continue to get “smarter” over time with the benefit of more part files being added to the database as well as expanding partnership with other organizations. A recently announced partnership with ValueChain, for instance, will allow PrintSyst to access ERP data; through this partnership production results can be automatically fed to back into the database, “closing the loop of understanding,” Yona says.
I recently got a demo of PrintSyst Perfecter via GoToMeeting. To see how the software evaluates parts and issues recommendations in real time, watch the video below.
Resources and links
- Why additive manufacturing needs machine learning and AI
- An example of adaptive manufacturing based on AI that allows printers to self-correct during the 3D printing process
- More on machine learning
- More in our software video series
Transcript
Stephanie Hendrixson, Additive Manufacturing Media
I'm Stephanie Hendrixson with Additive Manufacturing Media. Adopting AM can be a slow process, especially for an established manufacturer that might have hundreds of different parts to evaluate individually for 3D printing. Even an experienced engineer is going to need time to decide whether and how to 3D print a given part. Luckily, there are software solutions based on machine learning and artificial intelligence that can help companies make those assessments more quickly, and have a better chance of successful prints. We'll look at one example in this video, part of our series on software shaping AM. Joining me is Eitan Yona, the cofounder of PrintSyst, a company that provides cloud-based software to help automate that decision-making process. Eitan, welcome. Tell me a little bit more about PrintSyst.
Eitan Yona, PrintSyst
Hey, so first of all, thank you for your time, I appreciate it. So my name is Eitan, and I'm the cofounder and VP product of PrintSyst. PrintSyst is all about 3D printing with confidence. We are bridging the gap between manufacturers to designers by using our artificial intelligence proprietary engine. And we're doing by providing a full analysis about 3D parts and providing suggestions for manufacturing methods in terms of AM technologies, also materials and also can show what are the potential failure points. All of that are done automatically super fast using artificial intelligence technology.
Stephanie Hendrixson
So something I find really interesting about your software is that it's not just giving you a yes or no decision, it's actually helping you try to assess your chances of success within the printing process. Can you talk a little bit more about how the software works and how the algorithm is making the decision whether this particular design is going to translate into a successful 3D printed part?
Eitan Yona
What we're doing is actually we can figure out from zero to 100, how much the part is suitable for each technology when it comes to additive manufacturing. When you upload the parts to our software, we're looking at the geometries, comparing those geometries to our database, our database fooled with bars, which already printed using additive manufacturing. And we are looking for similarities in the geometries. The database is tagged already by which technology is the best to go with based on the functionality of the part. We're looking for those similarities, and we can determine what is the best way to manufacture it.
Stephanie Hendrixson
Okay, so let's dive into this. Can we see an example?
Eitan Yona
Sure, of course. So let's, for example, open a new project. So just click on this area. Now, I need to choose the parts, I want to upload to the software. Let's go for example, with these five parts. I'm clicking open. And now the second thing that comes up is that I need to name the project. So just for this demonstration, let's call it Stephanie.
I'm clicking on the OK button. Now what happens is that the engine starts to analyze the parts. So this tool uses artificial intelligence technology. Based on the similarities, it will tell me which technology works the best for the part that we uploaded. So now it's processed. And we can see in just a few seconds, it will start to pile up and we'll get three suggestions. It can be that a part is not printable, it can be printable, or it can be a warning. A warning means that it requires further attention from the engineer before he goes to the manufacturing. And when it comes to warning, I can show the engineer what are the potential failure points. Now, this really reduced the time between the designer and manufacturer. Because instead of making a lot of iterations, I can send him directly a report of what are the potential failure points, and then he can just can fix it on the CAD software. And instead of doing those iteration is just in seconds, you could add entirely and probably the report. Now we can see now the parts are starting to pile up. And it's working really super fast. It's nothing like we I'm talking something like one minute, and we already analyzed five parts.
Now, let's for example, and go for this bracket. This is actually coming from the automotive industry, one of our clients. And you can see directly that there are suggestions of what is the recommended technology, what is the recommended material, and also I can get an automated pricing for the part itself. So I'm just clicking on the parts. And now what happens is that I can see of course, the picture. And there are two things that are the things that really reflects the AI engine capabilities are in this chart. What happens here is that this is showing me the scale from zero to 100 how much the part is suitable for each technology. And this is based on the similarities. So if I hover this mark, I can see that how many parts the AI engine recommendation is based on. So this is the number of parts that you find the same in the database. And we have like 91% of succeeding with going with FDM.
But we have a manufacturability problem. And we can see that in this heat map. So we have a wall thickness problem and also in hole diameter. In the part itself. I can click on this part on this picture. And what happens is, just in a second, we will see the analysis of where the potential failure points in this part in a visual way that you actually can send that directly to the engineer. You can see here that the green means that those are areas which are printable. And the orange represents where are the potential fail points when it comes to wall thickness. And blue means two parts have holes which are too small for manufacturing. So if for example, I unmarked these areas, I can see directly those holes which are too small for manufacturing, and when it comes to the thickness, and just going right over here. So I can see the problems, which are a year on this side, this hole is too close to the wall is going here, here and also in the back of the part. And this in general. So I can send actually this report with the viewer directly to the designer, and reduce this time of iterations by explaining to him where are the potential failure points.
Now, you can see that the recommendation of the engine in the beginning was to go with FDM. And also ABS technology, of course, you can override this suggestion. And by overriding the suggestion, the engine starts to learn which kind of geometry works with which kind of technologies and also materials and this will be based on your preferences. Let's say for example, that you are not satisfied with the ABS decision on the part. So we have something that we called the material guide that you can play with those sliders, for example. And by playing with those and clicking for example, on submit, now the engine looks for me. What is the best material among all the materials that I have in my library? And based on that I can get a recommendation for material. So that way, also the engine learns that this kind of material works properly with this kind of technology.
Stephanie Hendrixson
So this is really interesting, because the engine is not just using that existing database of part geometries. It's learning from the choices of the designer, of the engineer, as far as what process might be preferred, what material properties might be needed. Just through the course of putting different parts in and playing with the options, you're kind of teaching the software to get smarter, right?
Eitan Yona
Yeah, yeah, exactly. And this is how it works. This is the core of technology itself.
Stephanie Hendrixson
So in the example of the bracket that we just looked at, there were some different some different choices, like there was a comparison between FDM and SLS technology, there was a recommended material.And so all of that is customizable, based on what the engineer actually has available. Can we so can we talk a little bit about what it looks like to set this up?
Eitan Yona
Yes, so it's really easy process. And at the beginning of the process, of course, we have some of the settings, the basic settings that we would need to apply. For example, which technologies you're using, or, for example, you need to have some tuning about wall thickness issues or pin diameter based on the machines that you have in the company. Based on those components, you calculate the pricing of the parts itself.
And now, in terms of analytics about the entire projects, we also have the dashboard. This actually accumulates with all the parts that have been added into the software, under my user, of course. So we can see for example, what is the leading technology among all the parts that have been uploaded? That way you will be able to even understand what is the CapEx you need to invest in. You can make smarter decisions in terms of investing in new machines. This is something that is software, we're able to make a smart decision, what is the total estimation of the entire parts, how many parts have been succeeded how many parts have been failed.
Stephanie Hendrixson
Yeah, so I can see how this would be really useful for maybe a big manufacturer that has a large catalog of parts that they're trying to evaluate, or for a service bureau that's just dealing with a big variety of different parts. But I'm curious, you know, what do you see from your customers? Where do you see the software having the biggest impact?
Eitan Yona
It's all about, in big companies, the knowledge management in the organization, because there's a lot of employees that are working with additive, they're coming and going. Based on the experience of the engine, it can learn the patterns of people and can help them in making a smart decision in their organization. In the end, in the future, it will accumulate with all the data and experience that people gain. People who will join the organization or people that want to use additive, they can be kind of an expert right away. It will really automate the entire process.
Stephanie Hendrixson
So software gets smarter, but it also helps people get smarter.
Eitan Yona
Yeah, it is.
Stephanie Hendrixson
Eitan, thank you for joining me.
Eitan Yona
Sure. Thank you very much. I appreciate that.
Stephanie Hendrixson
Thank you to everyone for watching. If you'd like to learn more about PrintSyst or other kinds of software advancing additive manufacturing, see the links in the video description. And for more on industrial 3D printing visit AdditiveManufacturing.media.
Related Content
Seurat: Speed Is How AM Competes Against Machining, Casting, Forging
“We don’t ask for DFAM first,” says CEO. A new Boston-area additive manufacturing factory will deliver high-volume metal part production at unit costs beating conventional processes.
Read More3D Printed Ceramic Mug: The Cool Parts Show #48
MadeXBinary applies additive manufacturing to clay. The company achieves automated production of aesthetically pleasing kitchenware through digital pottery.
Read More8 Cool Parts From RAPID+TCT 2022: The Cool Parts Show #46
AM parts for applications from automotive to aircraft to furniture, in materials including ceramic, foam, metal and copper-coated polymer.
Read MoreAdditive Manufacturing Production at Scale Reveals the Technology's Next Challenges: AM Radio #28
Seemingly small issues in 3D printing are becoming larger problems that need solutions as manufacturers advance into ongoing production and higher quantities with AM. Stephanie Hendrixson and Peter Zelinski discuss 6 of these challenges on AM Radio.
Read MoreRead Next
AI-Driven Adaptive Manufacturing Software Aims to Self-Correct 3D Printing (Includes Video)
The AI software platform Blacksmith from Markforged shares feedback from its cloud-connected fleet of printers to identify and correct distortions in a 3D-printed part.
Read MoreWith Machine Learning, We Will Skip Ahead 100 Years
Machine learning or AI will prove vital to the advance of AM. Computational power will enable additive to advance much faster than if it had been invented in an earlier time.
Read More3D Printing Brings Sustainability, Accessibility to Glass Manufacturing
Australian startup Maple Glass Printing has developed a process for extruding glass into artwork, lab implements and architectural elements. Along the way, the company has also found more efficient ways of recycling this material.
Read More