Senvol ML Enables New Approach for Material Allowables Development
Senvol demonstrates use of machine learning software to enable path for rapid development of material property allowables for additive manufacturing.
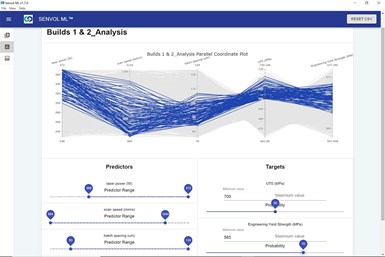
Senvol ML is a data-driven machine learning software to analyze relationships between additive manufacturing process parameters and material performance. Photo Credit: Senvol
Senvol recently demonstrated a machine learning approach to material property allowables development that the company says is more flexible, cost-effective and time-effective as well as just as accurate as the conventional approach (in this case, CMH-17) to allowables development.
The work was done as part of a contract awarded by America Makes, the national additive manufacturing institute, and funded by the U.S. Air Force, to apply its machine learning software, Senvol ML, to enable a path to rapid development of material property allowables for additive manufacturing (AM).
Senvol’s partners on the program included Northrop Grumman, the National Institute for Aviation Research (NIAR), Stratasys Direct Manufacturing and Pilgrim Consulting.
As part of the program, Senvol demonstrated a new approach to material property allowables development that leverages machine learning. The company says the machine learning approach is extremely flexible and able to handle any change to the AM process, which makes this approach well suited for sustainment in the long term. The program focused on demonstrating the approach using a Nylon 11 Flame Retardant material processed via a polymer powder bed fusion AM machine.
“Additive manufacturing is a modern and digital manufacturing method with rapidly tailorable processing. To continue to use traditional material allowables development approaches is a bottleneck to wider material and process options, and capabilities for additive manufacturing,” says Dr. Brandon Ribic, America Makes technology director. “Senvol’s program was very powerful in demonstrating an approach to additive manufacturing allowables that leverages the digital nature of the technology and leverages machine learning, a modern data analysis approach that has been shown to be extremely effective in a multitude of other industries.”
AM is starting to enable lightweight and rapidly produced designs that are revolutionary to various U.S. Air Force and commercial capabilities and applications. It is said these benefits cannot yet be fully realized due to the time and high cost of allowables development.
The high cost is said to stem, in large part, from the fact that material allowable development requires an enormous amount of empirical data to be generated, at a fixed processing point, meaning that all of the empirical data must typically be regenerated from scratch every time there is a major change in the process. This results in an AM process that is not only costly and time-consuming to implement the first time, but costly and time-consuming to maintain in the long-run when there are inevitably changes to the AM process.
The Senvol ML software supports the qualification of AM processes and was used in the program to develop statistically substantiated material properties analogous to material allowables. The company says it did so while simultaneously optimizing data generation requirements. It is said the software is flexible and can be applied to any AM process, AM machine and AM material.
“Senvol implemented data-driven machine learning technology that has the potential to substantially reduce the cost of material allowables development,” says Zach Simkin, Senvol president. “By demonstrating an entirely new – and significantly more efficient – approach to allowables development, Senvol aims to drive tremendous value for the U.S. Air Force, the America Makes membership and the additive manufacturing industry at large.”
Related Content
Additive Manufacturing Is Subtractive, Too: How CNC Machining Integrates With AM (Includes Video)
For Keselowski Advanced Manufacturing, succeeding with laser powder bed fusion as a production process means developing a machine shop that is responsive to, and moves at the pacing of, metal 3D printing.
Read More3D Printed NASA Thrust Chamber Assembly Combines Two Metal Processes: The Cool Parts Show #71
Laser powder bed fusion and directed energy deposition combine for an integrated multimetal rocket propulsion system that will save cost and time for NASA. The Cool Parts Show visits NASA’s Marshall Space Flight Center.
Read MoreBeehive Industries Is Going Big on Small-Scale Engines Made Through Additive Manufacturing
Backed by decades of experience in both aviation and additive, the company is now laser-focused on a single goal: developing, proving and scaling production of engines providing 5,000 lbs of thrust or less.
Read MoreHow Norsk Titanium Is Scaling Up AM Production — and Employment — in New York State
New opportunities for part production via the company’s forging-like additive process are coming from the aerospace industry as well as a different sector, the semiconductor industry.
Read MoreRead Next
3D Printed Polymer EOAT Increases Safety of Cobots
Contract manufacturer Anubis 3D applies polymer 3D printing processes to manufacture cobot tooling that is lightweight, smooth and safer for human interaction.
Read MoreProfilometry-Based Indentation Plastometry (PIP) as an Alternative to Standard Tensile Testing
UK-based Plastometrex offers a benchtop testing device utilizing PIP to quickly and easily analyze the yield strength, tensile strength and uniform elongation of samples and even printed parts. The solution is particularly useful for additive manufacturing.
Read MoreCrushable Lattices: The Lightweight Structures That Will Protect an Interplanetary Payload
NASA uses laser powder bed fusion plus chemical etching to create the lattice forms engineered to keep Mars rocks safe during a crash landing on Earth.
Read More